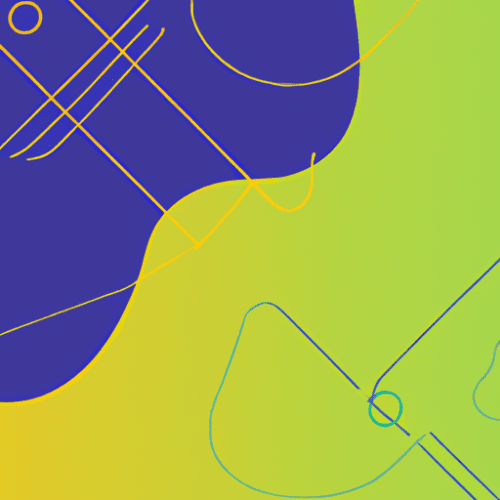
As digital ecosystems grow ever more complex, creating seamless, delightful user experiences is becoming increasingly challenging. Traditional UX design methods, while valuable, often rely on static user research, persona-driven assumptions, and manual optimizations. The advent of artificial intelligence (AI) and, more specifically, machine learning (ML) is disrupting UX conventions. Today, user journeys can be dynamically adapted in real-time, based on data-driven insights drawn from millions of granular user interactions.
In this article, we'll dive deep into how AI and machine learning are revolutionizing UX design. We'll explore key concepts, showcase impactful use cases, and discuss best practices and challenges for integrating AI into user-centered design. Whether you're a designer, developer, or product strategist, understanding AI-powered UX is crucial for shaping the future of digital experiences.
Main Research: How Machine Learning Is Transforming User Journeys
1. Personalization at Scale
One of the most significant advances of AI in UX is hyper-personalization. Unlike traditional segmentation, machine learning models can analyze massive datasets—including clicks, scrolls, purchase history, and even sentiment analysis—to tailor experiences to individual users.
- Content Recommendations: Platforms like Netflix and Spotify leverage collaborative filtering and deep learning to suggest movies, shows, and songs you’re likely to enjoy, making the journey more engaging and sticky.
- Dynamic Interfaces: E-commerce websites use AI to showcase personalized product assortments. The system learns which layouts, colors, and call-to-actions drive conversion for each user, optimizing the page in real time.
Research has shown that personalized user journeys can increase engagement metrics by up to 60% and conversion rates by as much as 20%. This kind of personalization simply isn’t feasible with manual, rule-based systems.
2. Predictive User Flows & Proactive Assistance
AI doesn’t just react to user actions—it predicts them. By analyzing context and behavior, ML models can forecast what users are likely to do next and proactively smooth their path. This predictive power translates into more intuitive, frictionless experiences.
- Intelligent Search and Autocomplete: Google’s auto-suggest, Amazon’s predictive search dropdowns, and intelligent chatbots anticipate queries before users finish typing, reducing cognitive load and guiding intent.
- Contextual Nudges: Banking apps may prompt users who regularly schedule payments to set up recurring transfers, or travel apps may suggest early check-in during a user’s travel day.
The result? Higher task completion rates, user satisfaction, and retention—key performance indicators for any UX team.
3. Accessibility and Inclusivity Improvements
AI-powered tools can significantly broaden accessibility in digital products. Machine learning models can transcribe audio to text for the hearing-impaired, automatically translate content, or adjust interface elements to accommodate visual or cognitive disabilities.
- Automatic Captioning & Voice Interfaces: Google Meet’s live captions and AI-powered voice assistants enable easier, more inclusive interactions for users with impairments.
- Adaptive UIs: AI can analyze how users interact (e.g., frequent zooming or slower reactions) and adjust font sizes or color contrast automatically, enhancing usability for everyone.
4. Data-Driven Design Decisions
Designers often struggle to interpret user data and translate it into actionable insights. Machine learning revolutionizes UX research by surfacing patterns, anomalies, and opportunities that would be invisible to human analysts.
- Automated A/B Testing: ML algorithms can rapidly iterate on button placements, colors, text, and layouts—testing thousands of combinations and zeroing in on what's most effective, far beyond traditional split-testing.
- User Feedback Analysis: Natural language processing (NLP) tools digest vast amounts of qualitative feedback from surveys, reviews, and social media to extract key pain points and satisfaction drivers.
This analytical power ensures that user journeys evolve based on real-world evidence, rather than intuition or limited samples.
5. Adaptive and Continuous Learning Systems
A unique strength of machine learning is its ongoing adaptability. AI-powered UX evolves over time, learning from new data, shifting user behavior, and external changes (like device or network conditions).
- Real-Time Error Handling: AI can detect user frustration signals (e.g., repeated failed login attempts) and proactively intervene with help dialogs, password reset links, or live chat support.
- Continuous Optimization: Instead of infrequent, large updates, designs can be tweaked continuously as ML models reveal new interaction patterns, keeping user journeys perpetually aligned with expectations and needs.
Challenges and Ethical Considerations
While AI-powered UX opens up new design vistas, it’s not without hurdles. Key challenges include:
- Privacy and Data Ethics: Hyper-personalization relies on vast data collection. Designers and developers must prioritize transparency, user consent, and responsible data stewardship.
- Algorithmic Bias: Biased models can exclude or misrepresent minority users, undermining inclusivity—a critical UX principle. Ongoing auditing and diverse datasets are essential.
- Loss of Human Touch: Over-reliance on automation can lead to generic or even uncanny experiences. Combining AI with empathetic, human-centric design ensures authenticity.
Addressing these issues requires multidisciplinary collaboration, continuous evaluation, and a strong focus on human values alongside technical innovation.
Conclusion: Designing the Future with AI-Powered UX
Machine learning is not just a technological upgrade—it’s a paradigm shift for user experience design. As we’ve seen, AI-driven systems can learn user preferences, anticipate needs, remove friction, and democratize accessibility in ways that static, manual design approaches never could.
For UX teams, the challenge—and opportunity—is to harness AI thoughtfully. Success lies in blending algorithmic intelligence with human insight, maintaining transparency, and relentlessly centering on user needs. AI-powered UX isn’t about eliminating designers, but empowering them to create richer, more adaptive journeys than ever before.
In the coming years, expect to see even more innovative applications of machine learning in UX, such as emotion-aware design, real-time adaptive storytelling, and ethical personalization. The future of user experience is not just data-driven—it’s learning-driven.
Are you ready to embrace the AI-powered UX revolution? Start small: identify a user journey pinch-point, explore how ML might address it, and iterate boldly. Your users—and your metrics—will thank you.